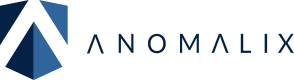
AI is quickly reshaping how businesses address digital identity. With the complexity of access control, compliance requirements, and cyberattacks rising, most businesses are turning to artificial intelligence to help them make more effective security decisions—quicker and with more context.
This whitepaper explores the intersection of AI and identity security. It outlines how AI is used to detect suspicious behavior, automate routine access decisions, and support security teams handling large volumes of identity information. It explains the risks associated with using machine learning models within such a delicate domain, including the rise of AI-driven attacks and concerns about transparency and fairness.
We provide practical recommendations for organizations seeking to use AI to handle Identity and Access Management (IAM). There are examples of common use cases, potential disadvantages, and a range of best practices to ensure you can integrate AI tools securely. Metrics for success—both technical and user-focused—are also outlined to support long-term measurement and improvement. Also discussed is a readiness checklist to help you plan your next steps responsibly.
Whether you're contemplating applying AI to automate identity governance or improve threat detection, this whitepaper gives an overview of what's possible, what's risky, and what's on the horizon.
Identity remains at the epicenter of user, system, and device engagement within today's digital landscapes. Every login, access request, or modification to permissions adds another layer to the growing stack of decisions organizations are faced with—many of which need to happen in real time. Managing this complexity has become harder, especially as hybrid work, cloud adoption, and regulatory demands increase the volume and variety of access-related activity.
Legacy IAM systems, while still required, were not designed to keep pace with this speed and volume. Many rely on static rules, manual reviews, or outdated policies that are not indicative of real behavior. That gap between how systems function and how human beings really do function introduces new threats—and opportunities.
Artificial intelligence offers a different solution. AI can help organizations react to potential threats sooner by examining vast amounts of data, identifying patterns in behavior, and flagging anomalies. For example, instead of based on the user's role or where they are, AI solutions can examine their general activity and whether a specific action is out of the ordinary for them.
At the same time, its use in identity governance raises concerns over trust, accountability, and control. What if the system refuses access to someone for no valid reason? Whose fault is it when the decision causes tension—or a data breach?
This white paper explores these questions through the lens of identity security. As we’ll see, AI in identity management isn’t just about automating decisions. It’s about building systems that can adapt, observe, and respond—without losing sight of accountability.
IAM solutions have traditionally used pre-defined rules. A user in department X gets access to certain tools. Permissions are applied or revoked on the basis of titles or approvals, all done by hand. These systems work—until they fail. As access needs get more fluid and threats grow in sophistication, static rules are not sufficient.
AI brings in flexibility. Instead of looking at roles or hierarchy alone, AI actually considers actual user behavior as well. It works with patterns, looks at the context, and uses historical data to make educated access decisions. For an organization managing hundreds or thousands of users, this makes all the difference.
One of AI’s most promising applications in IAM is anomaly detection. By monitoring login activity, device usage, time-of-day behavior, and more, AI systems build a baseline for each user. If something deviates—say, a sudden login from a foreign country or an unusual access request—the system can flag or block it automatically.
This behavior-based approach isn't all about security. It also reduces the level of alert fatigue. Instead of making noise, AI systems help triage real risk by determining what "normal" is for each identity.
In addition to access management, AI helps to streamline the identity lifecycle. From onboarding to offboarding, AI-based tools can suggest or assign permissions based on past behavior, job similarity, or collaboration. Administrators can still override these decisions, but the administrative overhead is cut down.
For example, when a new data analyst is brought onto a team, the system can automatically recommend access to the same dashboards and tools peers are working with—without waiting for a request. When an employee leaves, AI can ensure all permissions are removed promptly, closing the window for abuse.
In finance, AI systems help to identify insider fraud by highlighting suspicious access of customer data. In healthcare, they are able to monitor the way employees access sensitive patient information. Across sectors overall, organizations use AI to prevent threats but also to make faster, smarter identity decisions.
The common theme of these is context. AI provides more of it—enabling organizations to move away from assumption and toward fact-based decision-making.
As AI becomes more accessible, it is also being used by attackers to upgrade their own playbooks. Tactics previously only possible for researchers or giant tech companies are now being used to produce sophisticated phishing campaigns, develop deepfakes, and automate credential stuffing at scales and speeds that are hard to match.
One such new risk is the creation of synthetic identities—phony user profiles made of a mix of real and forged information. Synthetic identities might be used to open accounts, move money, or grant access to systems, and remain undetected because they do not resemble typical patterns. Text, pictures, and even audio clips created by AI can impersonate these profiles well enough to circumvent basic security measures.
AI is also being used to monitor and mimic user behavior. A bot that can learn about how an employee gains access to systems, when they log in, and what files they open can mimic that to remain undetected. These are not speculative threats. In the finance and healthcare sectors, for instance, attempts have already been made.
The majority of identity systems that use AI are basically black boxes. Decisions are made—access granted or denied—but the reasons why are hard to follow. That becomes a problem when a decision must be appealed or audited. It gets even more complicated when regulations require proof of how access decisions are made.
Firms that put too much trust in AI-powered access control risk creating a system that cannot be questioned. And when it denies customers or employees, on the basis of some unexplainable decision process, that erodes trust.
Explainability is not only a technical issue—it's also an issue of usability. Security personnel need to understand why AI systems made their conclusions, especially where those systems are making decisions about actual individuals.
Deploying AI in identity management is more than just implementing a new piece of software. It can demand specialist knowledge, which most teams don't have. Understanding machine learning models, data for training, and systems optimization for specific organizational needs all take time and knowledge.
Furthermore, most organizations are reliant on antiquated IAM systems. Migrating AI solutions onto legacy systems can lead to delay or complexity. With no plan in place, businesses may proceed without a plan—leading to fragmentation instead of security improvement.
Regulations like GDPR, CCPA, and others are getting stricter on data processing, and AI models usually require a lot of personal data to function effectively. If they are used without disclosure or consent, organizations can be fined.
There is also a growing expectation that AI-based decisions—especially identity and access ones—be equitable and unbiased. When a model discriminates or denies access in an incorrect manner, the reputational and legal consequences are potentially enormous.
The push to leverage AI for identity management has obvious advantages but introduces a new level of accountability. Balancing that is one of the biggest challenges organizations are grappling with today.
Before adopting AI tools for identity management, it’s essential to define who is responsible for overseeing how those tools are selected, implemented, and maintained. Data, compliance, and security groups must be involved in governance designs. Creating clear policies regarding data use, approvals for access, and auditing also helps avoid confusion in the future.
This is not just about meeting regulatory obligations. Governance gives organizations a framework for decision-making when AI output is unclear or in dispute. It also ensures that AI tools are directed towards broader business and ethical goals.
AI can speed up much of identity security work, but not every decision must be automated. Inserting checkpoints—especially for high-risk actions—is the most important aspect of accountability. For example, AI can flag suspicious activity, but it's a human being's responsibility to review the context before acting.
This hybrid approach fosters trust. It also puts organizations more in charge of how AI models are used and how their decisions are interpreted.
Zero Trust architecture presumes nothing about any user or device being trustworthy. Every request must be verified, every time. AI technologies can facilitate this model by tracking behavior in real time and adjusting access by risk level.
Instead of relying on a fixed set of credentials or device IDs, AI systems analyze the entire context: time of access, location, behavior patterns, etc. If something seems out of place, access can be blocked or tagged for further examination. This makes Zero Trust more dynamic and responsive.
Traditional IAM solutions are likely to rely on scheduled checks of access permissions. AI allows identity behavior to be tracked in near-real-time. What this means is abnormal patterns are caught early, shortening the gap between discovery and reaction.
Ongoing monitoring is especially useful in the identification of inactive accounts, permission creep, or insider threats. AI capabilities can raise these matters to the surface before they become security events.
Not all AI software is created equally. Before picking a solution, organizations should learn about how the model was trained, how the decisions are reached, and the type of data it draws on. Vendors need to disclose these elements clearly.
It's also good to ask about what happens in cases of error. Can AI choices be overridden by administrators? Do logs exist to enable auditing? Can the system provide explanations about why a given user was denied access or marked for monitoring?
Choosing a tool that is transparent, flexible, and controllable guarantees it's within your company's risk tolerance.
Finally, identity security is more than prevention and detection. When AI is involved, businesses need a plan for when things inevitably go wrong. That includes having written procedures, audit trails, and an explicit process for remediation of errors—whether by the AI system or the users.
A thoughtful approach to AI-led identity security starts with planning, moves on to wise implementation, and grows with regular feedback. It is not perfection—it's creating a system that can evolve and become better day by day.
The adoption of AI in identity and access management is also often framed as a technical benefit. However, without benchmarks set, it becomes hard to understand if the transformation is actually improving security, effectiveness, or the user experience. The setting of milestones beforehand—and continuously tracking them—is what allows organizations to know where AI is taking effect and where expectations may be adjusted accordingly.
One of the most concrete benefits of AI-driven identity systems is quicker detection of threats. Monitoring the latency in identifying and acting on suspicious access behavior can be used to gauge the performance of AI models in real-world scenarios. Reductions in detection time usually indicate improved threat visibility, particularly if AI is optimized for detecting subtle anomalies.
A system that produces too many false positives can swamp security teams. A system that does not detect actual threats might impose unacceptable risks. Keeping an eye on both types of errors—and how they trend—gives a sense of the model's accuracy. If either metric rises, it's probably time to retrain the model or adjust thresholds.
AI tools should limit the number of access decisions that require humans to act. Monitor the amount of provisioning or deprovisioning in auto versus manual mode. A steadily reduced workload means the system is learning and applying patterns for access.
Tracking how quickly identity-related requests—such as changes in access or approvals—are handled before and after implementing AI can signify gains in productivity. Better turnaround time allows for more productivity and less user frustration, especially in large or dispersed teams.
AI platforms that support automated logging, traceability of decisions, and risk-based reporting can aid organizations during audit readiness. Teams should track the amount of time spent in creating IAM audit reports, along with the number of times identity records are found to be outdated or incomplete.
While technical statistics are important, user experience is important too. User feedback channels or regular surveys can determine if AI IAM systems are creating confusion or assisting in simplifying access. An increase in frustration levels might indicate usability or transparency problems with the system.
Emphasis on these kinds of metrics offers a better overall view of AI IAM performance—going beyond jargon and into tangible results.
It is wise to evaluate if your organization is prepared—technically, operationally, and strategically—to introduce AI in your identity security infrastructure. The checklist below can guide teams in noting possible gaps, prioritizing planning activities, and building the foundation for responsible AI adoption.
Plot out your existing tools, data sources, and processes for identity management. This encompasses onboarding, access approvals, regular reviews, and offboarding. Understanding where decisions occur—and who is accountable—makes it simpler to determine where AI might bring value.
Look for daily IAM patterns. Are there workflows clogging up the team? Same decisions made for the same thing? These are good prospects to automate or support with AI.
Any AI implementation that handles personal or behavioral data needs to have legal and compliance stakeholders involved early on. Get your strategy in sync with regulations like GDPR, CCPA, or HIPAA to avoid risk down the line.
Identify what you want to do with AI in IAM. Faster response times? Reduced manual review? Enhanced anomaly detection? Establishing baseline metrics at the outset will enable you to track progress and adjust your strategy subsequently.
Evaluate your team's current experience with AI, machine learning, or analytics programs. If skills are lacking, plan to train or consider hiring external partners who specialize in deploying AI.
Determine who will monitor the AI system performance, make access decisions, and monitor suspicious activity. AI software can make decisions automatically, but humans need to guide and approve key results.
Ask vendors to provide their explainability features, model training methodologies, data requirements, and integration possibilities. Think about whether or not the solution will scale with your company's requirements—not tomorrow, but tomorrow.
Thoughtful planning will allow your AI identity security strategy to be achievable, measurable, and aligned with your risk profile.
AI is changing the way organizations do identity security. It offers new methods for threat detection, access granting, and risk management for increasingly complex systems. But with these new possibilities come new questions—of control, of trust, and of longevity.
For most, the question isn't whether to use AI in identity management—but how to do it responsibly. The organizations that are getting the most out of AI aren't just adopting the latest tools. They're designing systems that can explain decisions, adapt to new threats, and help the people who use them every day.
As identity security continues to mature, a number of trends are appearing. Predictive models are beginning to identify identity risks before they arise. Generative AI is being tested for automating help desk operations, user provisioning, and documentation. Regulations, meanwhile, are catching up, and organizations will need to demonstrate how their systems work—and who's accountable.
For organizations starting from the beginning, it's best to step back and review current IAM processes. Where are the manual choke points? Which decisions are repetitive and predictable? Where is visibility inadequate? These are generally the most apparent places to start with AI-powered augmentations.
Finally, success in this field is dependent as much on people and processes as it is on technology. Those organizations that create the most effective identity systems will be those that balance automation with review, speed with examination, and innovation with accountability.